The Importance of Data Labeling in Modern Business
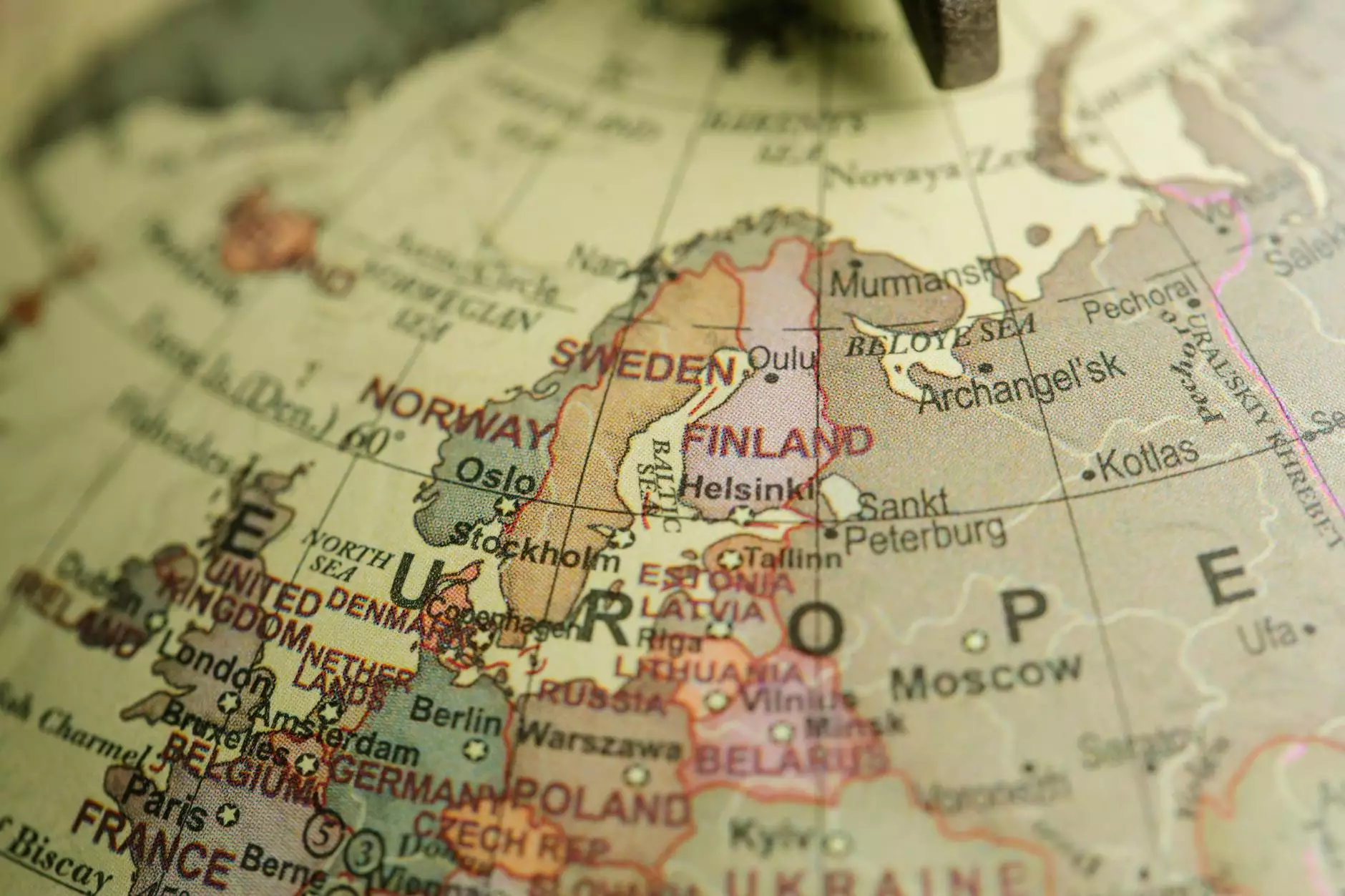
Understanding Data Labeling
Data labeling is the process of tagging or annotating data, so that machines and algorithms can better interpret, classify, and utilize this information. This critical task forms the backbone of various applications in machine learning (ML) and artificial intelligence (AI).
In essence, data labeling transforms raw data into a structured format that provides context and meaning, enabling machines to make informed predictions and decisions. Without accurate data labeling, the advancements in AI and ML would be significantly hindered.
The Role of Data Annotation Tools
Data annotation tools are essential in the data labeling process, providing the necessary functionalities to efficiently tag and manage large datasets. These tools facilitate the following:
- Efficiency: Automating various aspects of the labeling process speeds up data preparation.
- Accuracy: High-quality tools reduce human error through guided interfaces.
- Collaboration: Many platforms allow teams to work together seamlessly, improving workflow.
- Scalability: Tools can handle massive datasets, essential for training robust AI models.
By harnessing powerful data annotation tools, businesses can ensure that their datasets are labeled consistently and accurately, which leads to more effective machine learning models.
Applications of Data Labeling in Various Industries
The applications of data labeling span numerous industries, each benefiting from tailored AI solutions.
1. Healthcare
In the healthcare sector, data labeling is critical for various applications, including:
- Image Recognition: Annotating medical imaging data to assist in diagnosing diseases.
- Electronic Health Records: Categorizing patient data for better management and predictive analytics.
2. Autonomous Vehicles
For autonomous vehicles, data labeling is vital for:
- Object Detection: Labeling images to help vehicles identify pedestrians, signs, and obstacles.
- Path Planning: Providing contextual information for better route navigation.
3. Retail
In retail, data labeling assists with:
- Sentiment Analysis: Tagging customer feedback to improve services and product offerings.
- Inventory Management: Annotating product data for enhanced recommendations and stock management.
Building a Robust Data Annotation Platform
A strong data annotation platform combines cutting-edge technology with user-friendly interfaces to streamline the data labeling process.
Key features of an excellent data annotation platform include:
- Variety of Annotation Types: Supporting multiple types of annotations, such as bounding boxes, polygons, and semantic segmentation.
- Real-Time Collaboration: Allowing multiple users to work on the same dataset simultaneously.
- Integration with Machine Learning Pipelines: Seamlessly connecting with existing ML workflows to expedite processes.
- Quality Control Mechanisms: Implementing review processes to ensure the highest quality of labeled data.
KeyLabs.ai offers an advanced data annotation platform designed to meet the needs of businesses across multiple sectors, ensuring that data is labeled accurately and efficiently.
The Importance of Quality in Data Labeling
Quality is paramount in data labeling because the performance of machine learning models heavily relies on the quality of the data they are trained on. High-quality, well-labeled data leads to more reliable outputs, whereas poorly labeled data can result in inaccurate predictions.
Businesses must implement stringent quality control measures, such as:
- Regular Audits: Conducting periodic checks on labeled data to identify errors.
- Feedback Loops: Establishing systems for continuous improvement based on model performance insights.
- Training Programs: Implementing thorough training for labelers to minimize errors.
Challenges in Data Labeling
Despite its significance, data labeling comes with numerous challenges that businesses must navigate:
- Scalability: As datasets grow, maintaining consistency and accuracy becomes challenging.
- Cost: High-quality data labeling can be resource-intensive, demanding significant investments in time and finance.
- Complex Data Types: Handling unstructured or complex data, including nuanced audio and video data, can impede labeling efforts.
Addressing these challenges requires a combination of advanced technology, skilled labor, and effective management strategies to streamline the labeling processes.
Future Trends in Data Labeling
The future of data labeling is promising, with emerging trends that are shaping its evolution:
- AI-Assisted Labeling: Utilizing AI to assist human annotators can enhance efficiency and accuracy.
- Crowdsourcing: Leveraging larger crowds to perform less complex labeling tasks can distribute workload and reduce costs.
- Automated Annotation: Innovations in machine learning may enable more automated systems for data labeling, significantly reducing the time required.
Adopting these trends will provide businesses with a competitive edge in data-driven decision-making and machine learning applications.
Conclusion
In conclusion, data labeling is a vital component of the modern business landscape, influencing a wide range of industries and applications. By investing in robust data annotation tools and platforms, companies can effectively harness the power of their data to drive innovations and achieve strategic goals. Emphasizing quality in the labeling process is critical to the success of machine learning initiatives, ultimately leading to improved business outcomes.
KeyLabs.ai stands at the forefront of this evolution, providing businesses with comprehensive data annotation solutions tailored to meet specific needs. By adopting effective data labeling strategies, companies can unlock the full potential of their data-driven initiatives.