Understanding the Importance of Bounding Box in Data Annotation
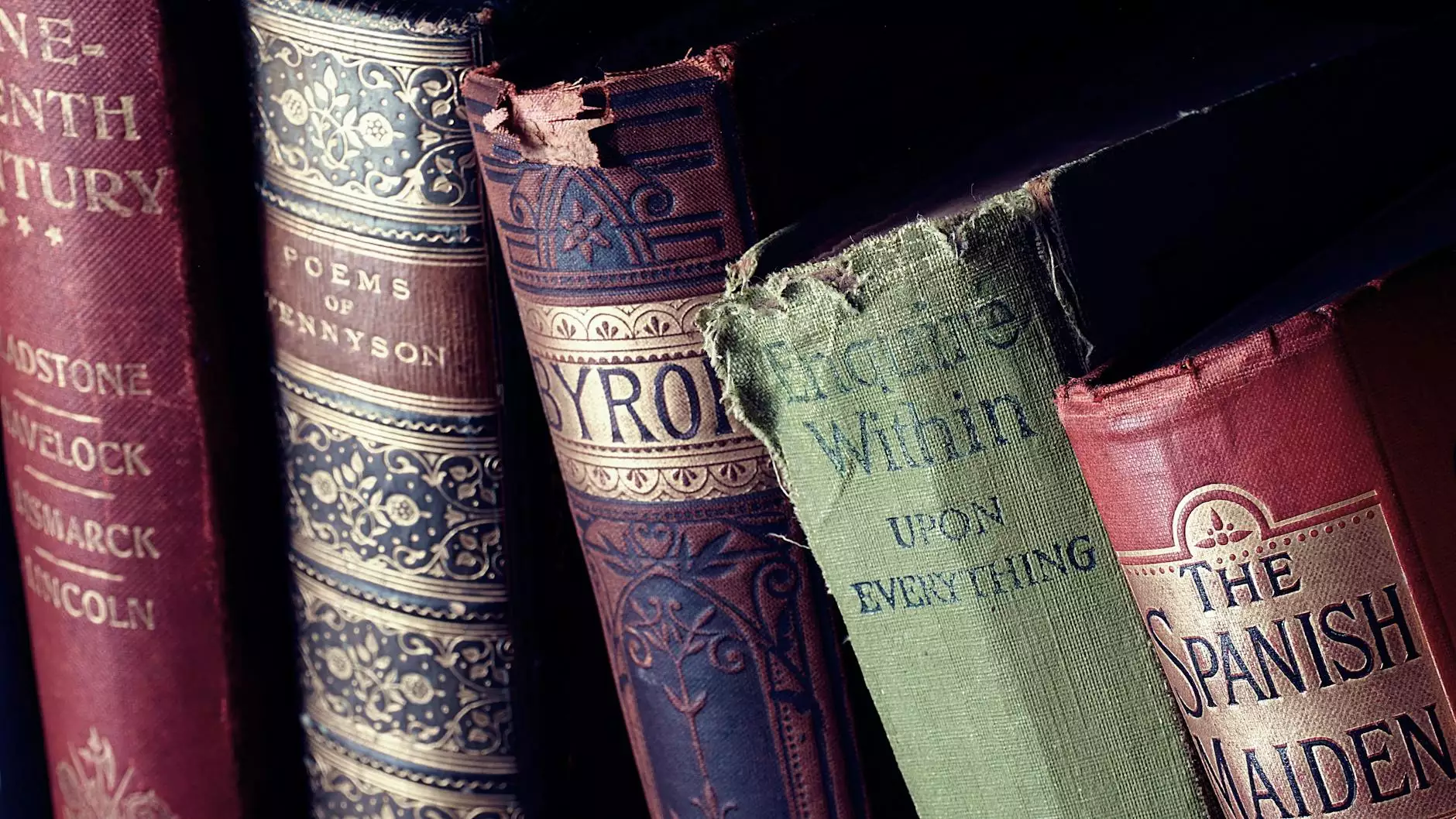
In the realm of data annotation, especially for applications involving machine learning and computer vision, the term "bounding box" is pivotal. This article delves into the significance of bounding boxes, their applications, and their essential role in enhancing data quality for AI and ML training.
The Concept of Bounding Box
A bounding box is defined as a rectangular box that is used to outline an object within an image. This concept may seem straightforward, but its implications in the field of data annotation are profound. By accurately defining the location of an object in an image, bounding boxes allow machine learning models to understand the spatial context and relationships of various objects.
Typical Applications of Bounding Boxes
Bounding boxes find extensive usage across various industries. Here are some primary applications:
- Autonomous Vehicles: In self-driving technology, bounding boxes help identify and track pedestrians, cyclists, and other vehicles to improve safety and navigation.
- Surveillance Systems: By outlining suspicious activities or movements, bounding boxes enhance the effectiveness of security systems.
- Healthcare Imaging: In medical imaging, bounding boxes can help healthcare professionals identify and assess tumors, lesions, or other abnormalities within diagnostic imagery.
- Retail Analytics: Shops use bounding boxes in video feeds to understand customer behavior and optimize layouts based on shopping patterns.
The Role of Bounding Boxes in Machine Learning
For machine learning models, especially those related to image recognition, the accuracy and precision of bounding boxes directly correlate with the model's performance. Here are key ways bounding boxes enhance machine learning efforts:
1. Training Data Optimization
Bounding boxes provide essential labels that aid in training algorithms effectively. By annotating images with bounding boxes, you empower machine learning models to learn where specific objects are located, improving their object detection capabilities significantly.
2. Enhancing Model Precision
When a model correctly identifies objects based on bounding boxes, it increases precision in recognizing similar objects in new, unseen data. This capability is critical in real-world applications where accuracy is paramount.
3. Supporting Semantic Segmentation
Bounding boxes are often used in conjunction with semantic segmentation, where the focus is not only on the objects but on identifying pixel-level details. The bounding box serves as a guide, simplifying the segmentation process.
4. Enabling Transfer Learning
Bounding boxes also assist models that utilize transfer learning techniques. Pre-trained models can transition to new tasks more effectively when provided with robust bounding box annotations from the source data.
Choosing the Right Data Annotation Tool
Selecting an appropriate data annotation tool is crucial for effectively implementing bounding boxes in your projects. KeyLabs.AI offers cutting-edge data annotation tools designed to streamline the process, ensuring high-quality annotations.
Features to Look For
When choosing a data annotation platform for bounding box tasks, consider the following features:
- User-Friendly Interface: A well-designed UI enables annotators to work efficiently, minimizing training time and errors.
- Support for Multiple Annotation Types: Look for tools that allow not just bounding boxes, but also other annotation types like polygons and keypoints.
- Collaboration Features: Real-time collaboration capabilities enhance workflow when teams are annotating data across different locations.
- AI-Assisted Annotation: Some modern tools incorporate AI to assist in creating bounding boxes, speeding up the annotation process.
Best Practices for Annotating with Bounding Boxes
Implementing effective bounding boxes requires adherence to best practices to ensure accuracy:
1. Precision in Placement
Bounding boxes should tightly fit the objects being annotated, avoiding excessive white space while encompassing the entirety of the object. This precision directly influences model accuracy.
2. Consistent Labeling
Consistency is crucial in bounding box annotations. Establish clear guidelines on how to label specific objects and follow them strictly throughout the dataset.
3. Quality Control
Regularly review annotated data to catch errors or inconsistencies early. Employ a feedback loop to improve future annotations based on past mistakes.
4. Utilize Hierarchical Labels
When applicable, use hierarchical labeling within bounding boxes to provide more detailed insights into various aspects of each object, such as category or state.
Conclusion: The Future of Bounding Box Annotation
As artificial intelligence and machine learning continue to evolve, the significance of precise data annotation cannot be overstated. The bounding box remains a foundational element in object detection algorithms, providing the essential structure needed for advanced model training.
By utilizing sophisticated tools like those offered by KeyLabs.AI, organizations can enhance their data annotation efforts, ensuring they remain at the forefront of innovation. Through effective use of the bounding box technique, businesses can unlock new capabilities in data-driven decision making.
Explore KeyLabs.AI for Advanced Data Annotation
For businesses looking to streamline their data annotation processes and utilize powerful bounding box techniques, KeyLabs.AI offers state-of-the-art solutions. Empower your machine learning projects with high-quality annotated data and watch your AI applications thrive!